Top 20 Examples of How Vector Databases Enhance CRM Data
Content:
- Introduction
- Understanding Vector Databases
- Personalization through Behavioral and Preference Vectors
- Top 20 Examples of How Vector Databases Enhance CRM Data
Understanding Vector Databases
At its core, a vector database stores information in a format that machines can interpret more naturally – as Vectors. These Vectors are arrays of numbers representing various attributes and behaviors of customers.
The magic of Vector Databases lies in their ability to process and analyze these Vectors to identify patterns, similarities, and differences among customer profiles at an unprecedented scale and speed.
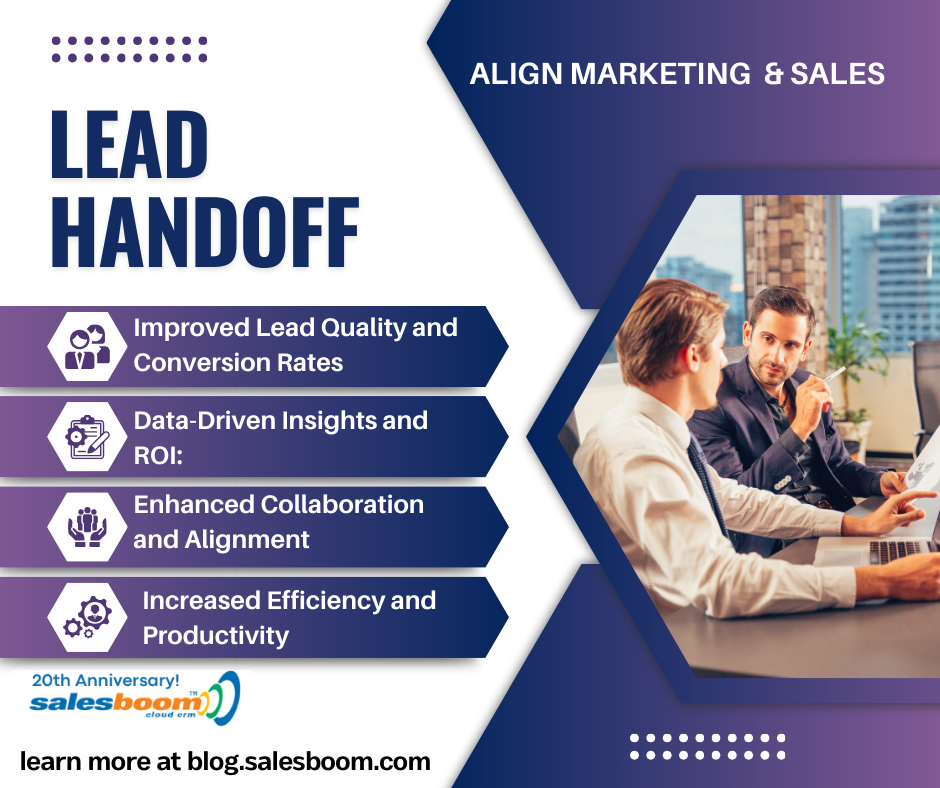
Personalization through Behavioral and Preference Vectors
Converting Data into Vectors
Every interaction a customer has with your brand, whether it's a purchase, a click on your website, or even a social media engagement, contributes to a comprehensive profile.
By analyzing this data, machine learning algorithms can convert these interactions into behavioral and preference Vectors – essentially, numerical representations of what customers want and how they behave.
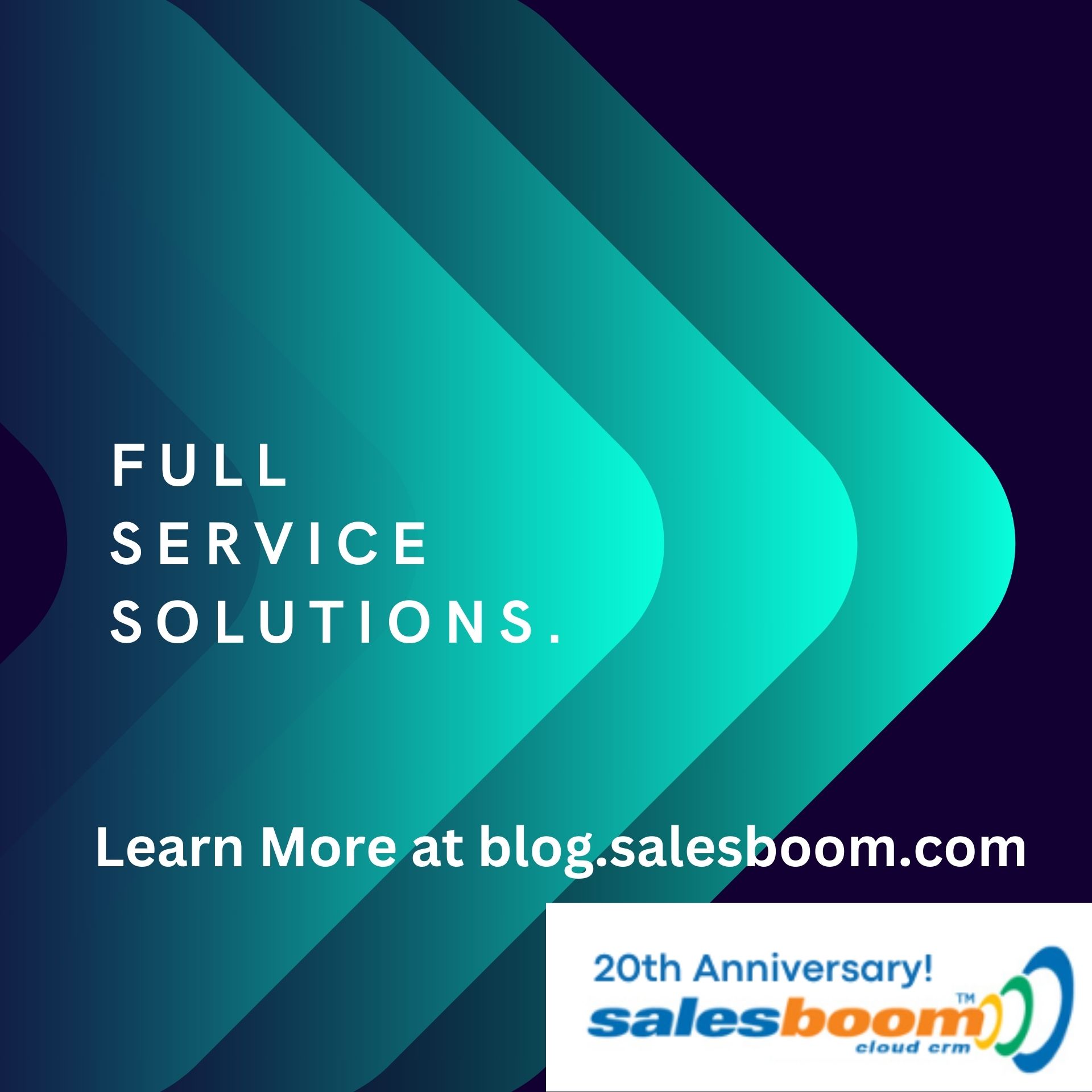
Discovering Similarities
These Vectors enable businesses to analyze and compare customer profiles on a much more nuanced level than traditional demographic-based segmentation.
For example, two customers in different age groups and geographic locations might have strikingly similar preference Vectors, indicating they might respond similarly to the same marketing message or product recommendation.
Top 20 Examples of How Vector Databases Enhance CRM Data
-
Personalized Customer Experiences:
By converting customer data into Vectors, companies can offer hyper-personalized experiences based on behavioral and preference similarities.
-
Predictive Customer Segmentation:
Using Vector Databases to analyze customer traits and behaviors for dynamic, real-time segmentation, beyond static categorization.
-
Enhanced Lead Scoring:
Implementing vector-based scoring systems that align prospective leads against ideal customer profiles for more accurate prioritization.
-
Churn Prediction:
Vectorizing customer activity and feedback to identify patterns indicating potential churn, allowing for proactive retention strategies.
-
Sentiment Analysis:
Analyzing customer feedback, reviews, and social media comments through Vector Databases to gauge overall sentiment and satisfaction.
-
Product Recommendation Systems:
Creating accurate recommendation engines by comparing customer preference Vectors with product or service Vectors.
-
Optimized Marketing Campaigns:
Using customer Vectors to identify the most responsive audience segments for targeted marketing campaigns.
-
Automated Support Ticket Routing:
Vectorizing support tickets and routing them to the most appropriate department or personnel based on issue similarity.
-
Market Trend Analysis:
Monitoring and analyzing vectorized social media and market data to quickly adapt to emerging trends.
-
Customer Lifetime Value Prediction:
Assessing the potential lifetime value of customers by analyzing their transaction and interaction Vectors.
-
Fraud Detection:
Identifying unusual patterns in customer transaction Vectors to detect and prevent fraudulent activities.
-
Dynamic Pricing Strategies:
Adjusting prices by analyzing customer purchase patterns and sensitivity Vectors.
-
Customer Feedback Analysis:
Vectorizing customer feedback for deeper insights into preferences, satisfaction levels, and potential improvement areas.
-
B2B Customer Management:
Segmenting and managing B2B clients more effectively by vectorizing organizational characteristics and interactions.
-
Content Optimization:
Adjusting marketing content based on customer engagement Vectors to enhance relevance and effectiveness.
-
Event-Based Marketing Triggering:
Using changes in customer behavior Vectors to trigger specific marketing actions or communications.
-
Sales Forecasting:
Improving accuracy of sales forecasts by analyzing historical sales data Vectors alongside current market trends.
-
Real-time Customer Interaction Tracking:
Monitoring and responding to customer interactions across various channels in real time, using vector similarity for immediate context understanding.
-
Cross-Selling and Upselling Opportunities:
Identifying the most relevant additional products or services for customers based on their historical purchase and preference Vectors.
-
Network Analysis for Influencer Marketing:
Identifying key influencers and decision-makers within a customer database by analyzing the Vectors of social connections, interaction patterns, and influence metrics.
These examples illustrate the breadth of applications for Vector Databases in enhancing CRM data, enabling businesses to not just understand their customers better, but also to predict behaviors, personalize experiences, and streamline operations in real-time.