Product Recommendation Systems: Revolutionizing Choices with Vector Databases
Content:
- Introduction
- Customizing Shopping Experiences in Online Retail
- Curating Content in Streaming Services
- Enhancing User Experience in Music Streaming
- Personalizing Literature Recommendations in Online Bookstores
- Tailoring Travel Recommendations in Tourism
In the competitive world of retail and online services, personalization is key.
Product recommendation systems have become a cornerstone of customer experience, and the incorporation of Vector Databases takes this to a new level.
By comparing customer preference Vectors with product or service Vectors, businesses can create highly accurate, tailored recommendation engines.
This approach not only enhances user experience but also drives sales and customer loyalty.
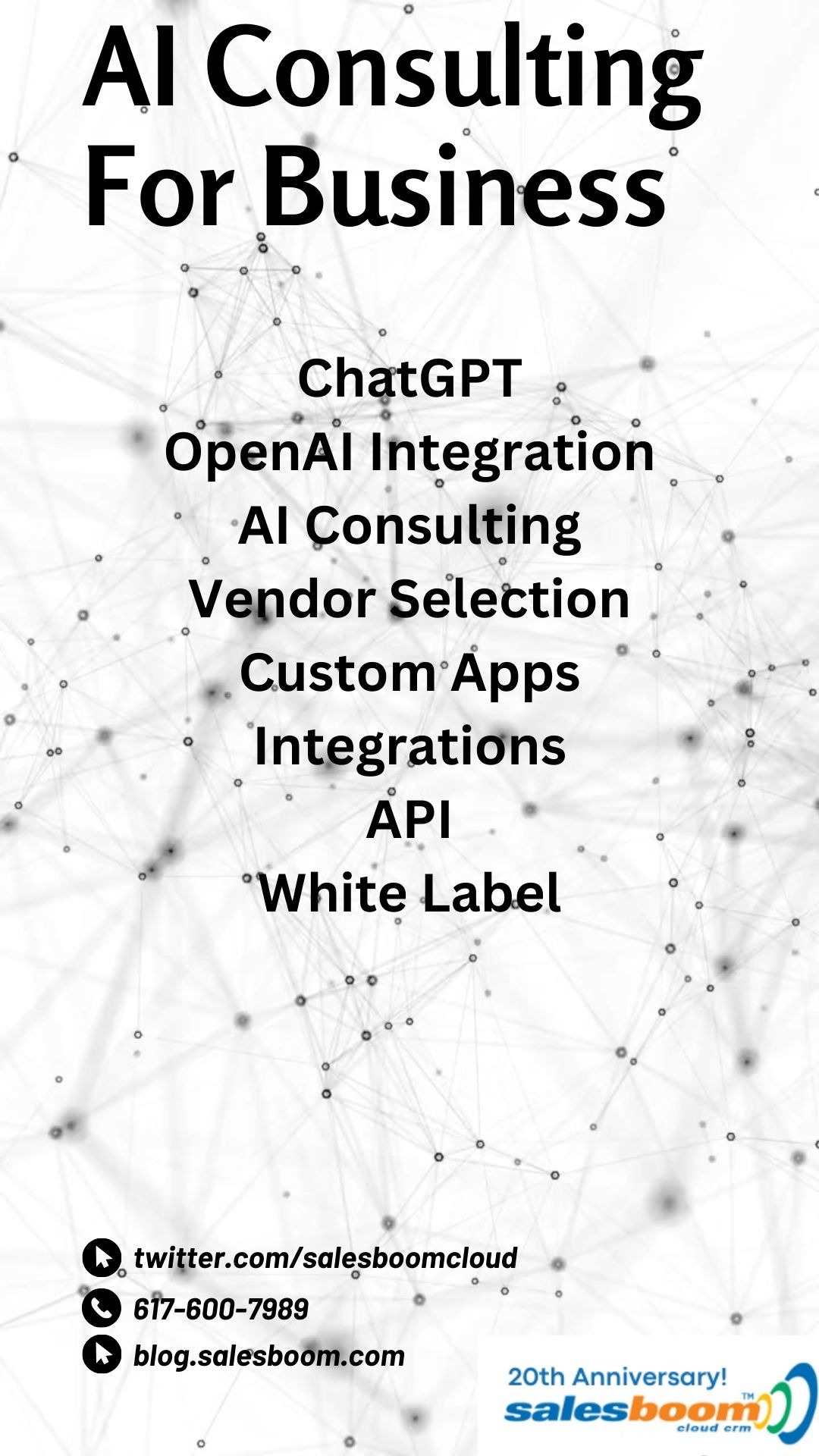
Let’s explore how various sectors can harness this capability.
-
Customizing Shopping Experiences in Online Retail
Scenario
An online retail giant aims to personalize shopping experiences for each user.
Application
Vector Databases can analyze historical purchase data, browsing patterns, and customer ratings to create preference Vectors for each customer.
Simultaneously, product Vectors are generated based on attributes like category, brand, price, and previous customer feedback.
By comparing these Vectors, the recommendation system suggests products that align closely with individual user preferences, thereby increasing the likelihood of purchase and enhancing customer satisfaction.
-
Curating Content in Streaming Services
Scenario
A video streaming service endeavors to keep viewers engaged with relevant content.
Application
Here, Vector Databases help by creating user Vectors based on viewing history, search queries, and ratings.
Each show or movie also has its vector representing genres, director styles, cast, user ratings, and more.
The system recommends shows and movies by identifying and suggesting content with similar vector properties to those the viewer prefers, keeping the content engaging and relevant.
-
Enhancing User Experience in Music Streaming
Scenario
A music streaming app wants to provide personalized playlists to its users.
Application
By vectorizing user listening habits, favorite genres, and frequently played tracks, along with Vectors for songs and artists based on genre, rhythm, lyrics, and listener reviews, the app can offer playlists that are more likely to resonate with each user’s musical taste.
This personalization not only enhances user experience but can also expose listeners to new but similar genres, increasing time spent on the app.
-
Personalizing Literature Recommendations in Online Bookstores
Scenario
An online bookstore strives to recommend books that keep readers coming back for more.
Application
The bookstore uses Vector Databases to analyze reader preferences through their browsing history, past purchases, and book ratings.
Books are vectorized based on genre, author style, themes, and reader reviews.
Matching these Vectors allows the system to recommend new books that align with the reader's interests, potentially increasing sales and reader engagement.
-
Tailoring Travel Recommendations in Tourism
Scenario
A travel recommendation site aims to offer personalized travel suggestions.
Application
Travelers' preferences are vectorized based on their past travel experiences, searches, and reviews.
Destinations and travel packages are also vectorized, reflecting attributes like location, activities, budget, and previous traveler reviews.
Comparing these Vectors enables the site to suggest destinations and packages that closely align with the traveler's tastes and past enjoyable experiences.
Product recommendation systems powered by Vector Databases are reshaping how businesses approach customer engagement and sales.
By accurately understanding and matching customer preferences to product attributes, these systems not only drive better user experiences but also foster brand loyalty and revenue growth.
In an era where personalization is not just desired but expected, leveraging Vector Databases for product recommendations is not just a strategy; it's a necessity in staying ahead in the market.