Churn Prediction: Proactive Strategies Using Vectorized Customer Data
Content:
- Introduction
- Personalized Retention in Telecommunications
- Predictive Engagement in Online Retail
- Enhanced Experience in Banking and Finance
- Optimized Subscriptions in Streaming Services
- Dynamic Retention in SaaS Platforms
Understanding and predicting customer churn – when customers stop doing business with a company – is crucial for maintaining a sustainable business model.
Traditional models for predicting churn often rely on basic historical data, such as purchase frequency or customer service interactions.
However, vectorizing customer activity and feedback to identify churn patterns offers a more nuanced and proactive approach.
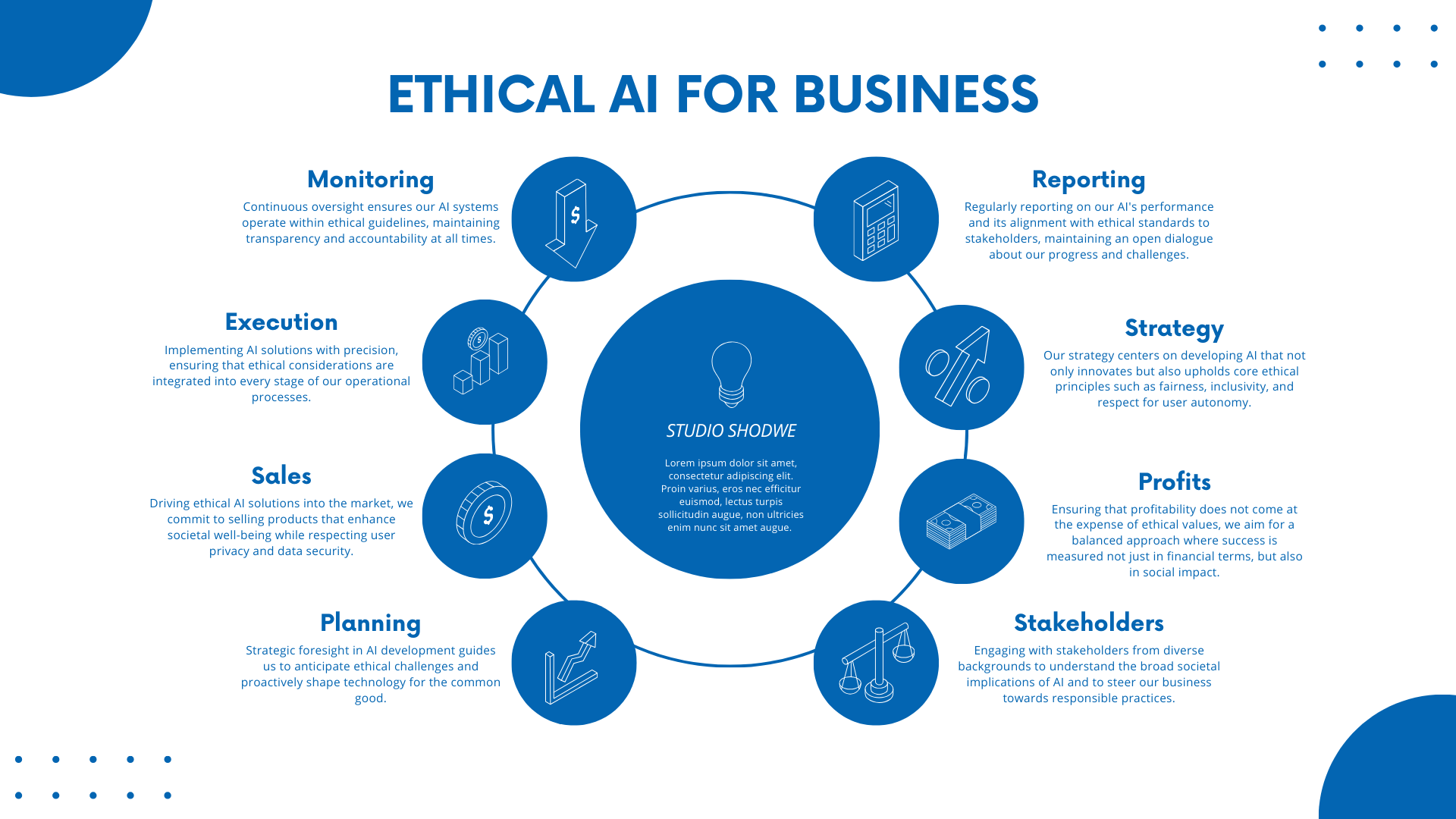
Let's delve into how businesses across various sectors can utilize vector-based churn prediction for effective retention strategies.
-
Personalized Retention in Telecommunications
-
Scenario
A telecom company faces high churn rates due to competitive market offerings and varying customer satisfaction levels.
-
Application
By vectorizing data such as call quality feedback, data usage patterns, customer service interactions, and billing history, the company can identify patterns indicating dissatisfaction or service issues.
Vectors that closely match those of past customers who churned can trigger personalized retention efforts, such as tailored plan upgrades, proactive customer service outreach, or special loyalty offers, directly addressing the issues leading to potential churn.
-
-
Predictive Engagement in Online Retail
-
Scenario
An online retailer struggles with retaining first-time buyers.
-
Application
Vectorizing customer behaviors — like browsing patterns, purchase history, and response to marketing campaigns — enables the retailer to recognize signs of waning interest.
Customers whose behavior Vectors resemble those who previously churned can be re-engaged through personalized recommendations, targeted discount offers, or loyalty programs, thereby increasing the likelihood of repeat purchases.
-
-
Enhanced Experience in Banking and Finance
-
Scenario
A bank wishes to reduce churn among its account holders and credit card users.
-
Application
Vectors created from transaction frequencies, types of services used, interaction with banking apps, and customer feedback can highlight clients potentially considering a switch.
Proactive measures, such as personalized financial advice, custom-tailored banking products, or improved customer service, can be deployed to retain these customers.
-
-
Optimized Subscriptions in Streaming Services
-
Scenario
A streaming service experiences a drop-off in user engagement and subscription renewals.
-
Application
By analyzing Vectors encompassing viewing habits, genre preferences, and session lengths, alongside customer service interaction quality and feedback, the service can identify subscribers at risk of churning.
Engaging these users with personalized content recommendations, exclusive previews, or subscription discounts can help in retaining them.
-
-
Dynamic Retention in SaaS Platforms
-
Scenario
A SaaS (Software as a Service) provider sees fluctuating renewal rates among its users.
-
Application
Vectorizing user interaction data with the software (feature usage, login frequency, support ticket history) along with satisfaction survey responses can reveal potential churn risks.
Offering targeted tutorials, feature updates, or personalized support can enhance user experience and loyalty, reducing the likelihood of churn.
-
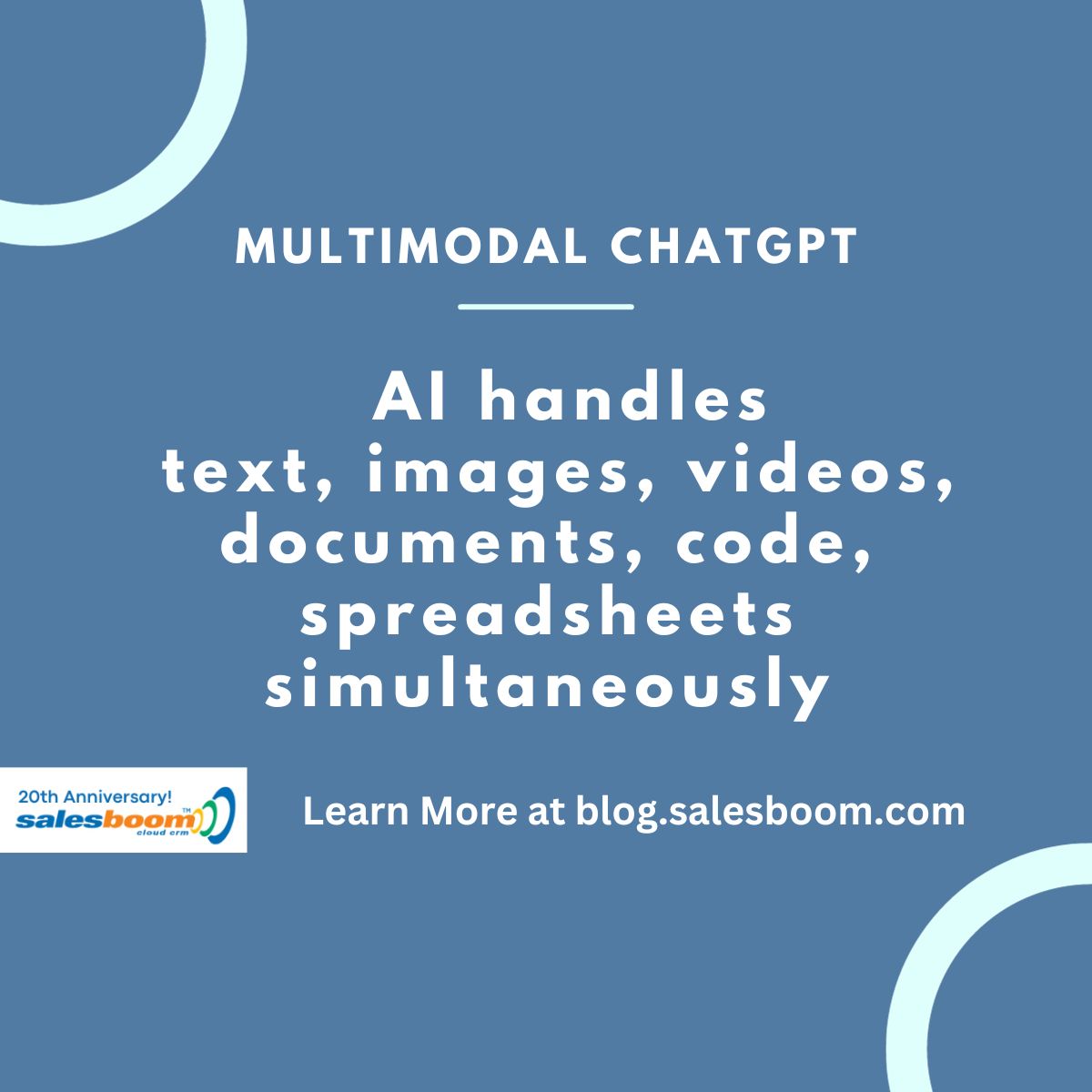
Churn prediction using vectorized customer data represents a significant advancement over traditional models.
This approach allows businesses to understand subtle shifts in customer behavior and sentiment, enabling them to take proactive, customized actions to retain customers.
As the business landscape becomes increasingly competitive and customer-centric, the ability to predict and prevent churn using advanced vectorization techniques will be invaluable in ensuring long-term customer satisfaction and loyalty.