Customer Lifetime Value Prediction - Maximizing Revenue Through Vector Analysis
Content:
- Introduction
- E-commerce: Tailoring Customer Experience
- Banking and Financial Services: Risk and Relationship Management
- Telecommunications: Enhancing Customer Retention
- Insurance: Personalized Policy Planning
- Retail: Streamlining Operations and Marketing
Understanding and predicting the Customer Lifetime Value (CLV) is a pivotal aspect of maintaining a sustainable business model.
With the integration of Vector Databases in analyzing customer transactions and interactions, businesses can gain deeper insights into the projected long-term value of each customer.
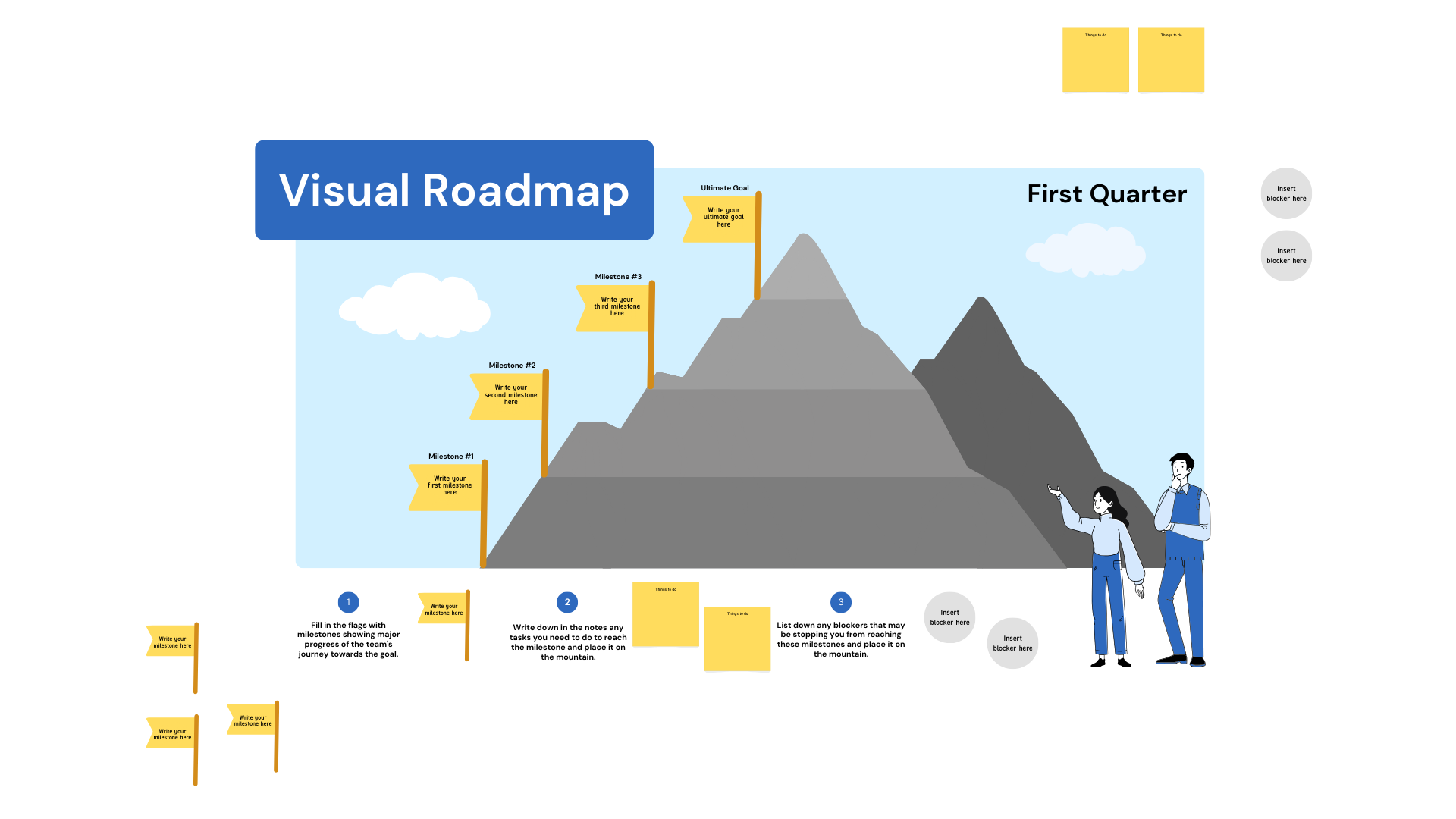
This approach facilitates more targeted and efficient resource allocation, marketing, and customer service strategies. Let's explore how different sectors can utilize CLV predictions using vector databases.
-
E-commerce: Tailoring Customer Experience
Scenario
An e-commerce business aims to optimize marketing spend and build lasting relationships with its most valuable customers.
Application
By vectorizing customer purchase history, browsing behavior, and review data, e-commerce platforms can accurately forecast the CLV of individual customers.
This insight allows for the customization of offers, loyalty programs, and recommendations based on predicted value.
It enables a more personalized shopping experience, encouraging repeat purchases and fostering long-term loyalty among high-value customers.
-
Banking and Financial Services: Risk and Relationship Management
Scenario
Banks and financial institutions seek to identify clients with the highest long-term profitability for prioritized relationship management and personalized financial product offerings.
Application
Vector analysis of transaction history, account balances, and interaction patterns with bank services can help in predicting the CLV of clients.
This prediction aids in personalizing banking services, aligning investment advice, and tailoring credit offers.
It can also guide risk management strategies by identifying clients with potentially higher lifetime value but requiring short-term credit support.
-
Telecommunications: Enhancing Customer Retention
Scenario
Telecommunication companies need to reduce churn and focus retention efforts on customers with high potential value.
Application
Through vectorizing call records, data usage patterns, customer service interactions, and payment history, telecom companies can forecast the CLV of their subscriber base.
Insights from this analysis can shape customer retention programs, bespoke data and calling plans, and customer service initiatives targeted at high-value segments, thereby enhancing overall customer satisfaction and reducing churn.
-
Insurance: Personalized Policy Planning
Scenario
Insurance companies want to optimize their policy offerings and customer service for clients who provide the most value over time.
Application
Vectorizing claim histories, policy amendments, and customer feedback, insurers can estimate the CLV of policyholders.
This enables them to offer tailored insurance packages, prioritize high-value customer claims and inquiries, and design loyalty programs, leading to improved customer retention and profitability.
-
Retail: Streamlining Operations and Marketing
Scenario
Retail chains aim to enhance in-store and online customer experiences for clients with the highest projected lifetime value.
Application
By analyzing Vectors from purchase data, customer service interactions, and loyalty program engagement, retailers can predict the CLV of their customer base.
This information is crucial for inventory management, store layout optimization, targeted marketing campaigns, and developing personalized shopping experiences, especially for customers who are more likely to be long-term, high-spenders.
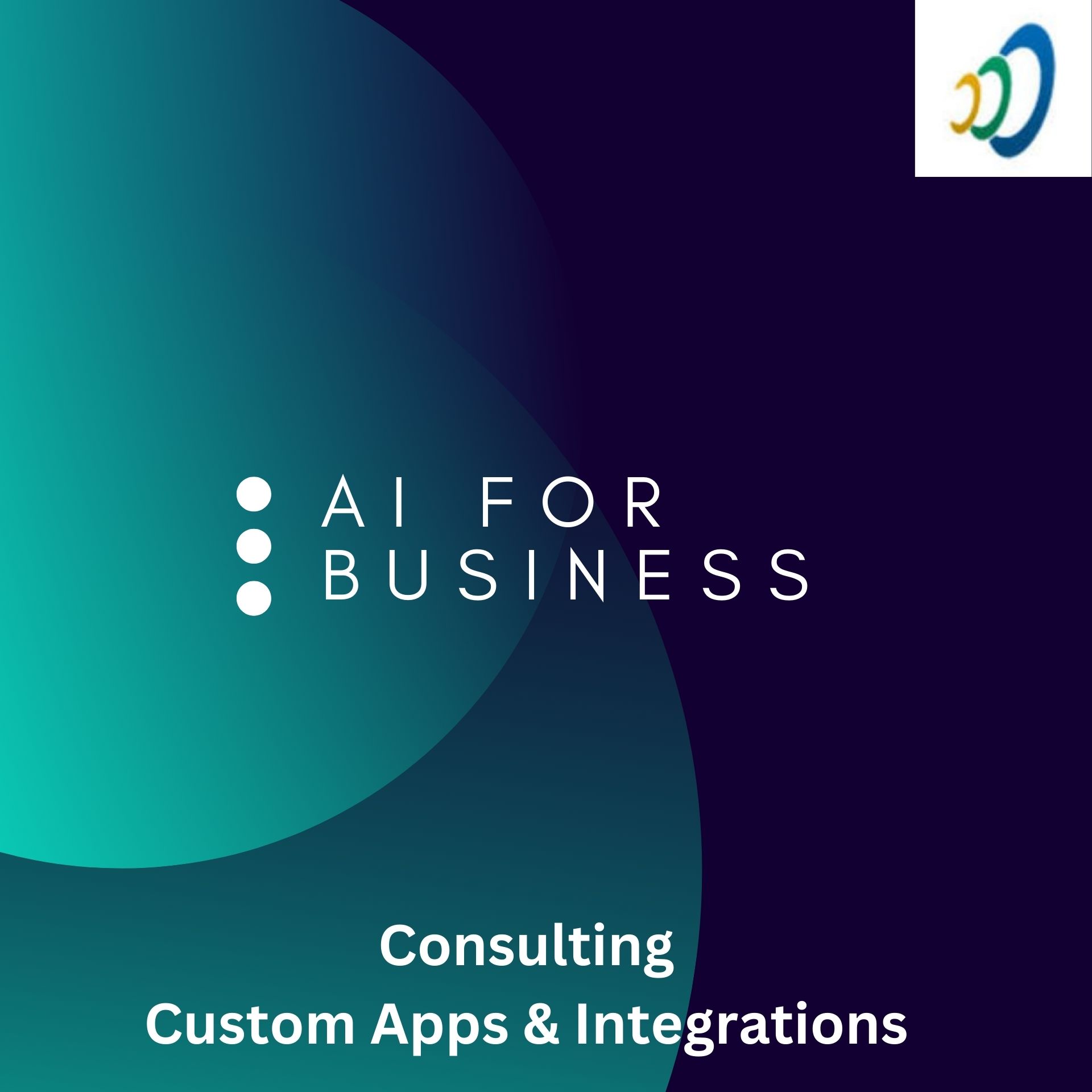
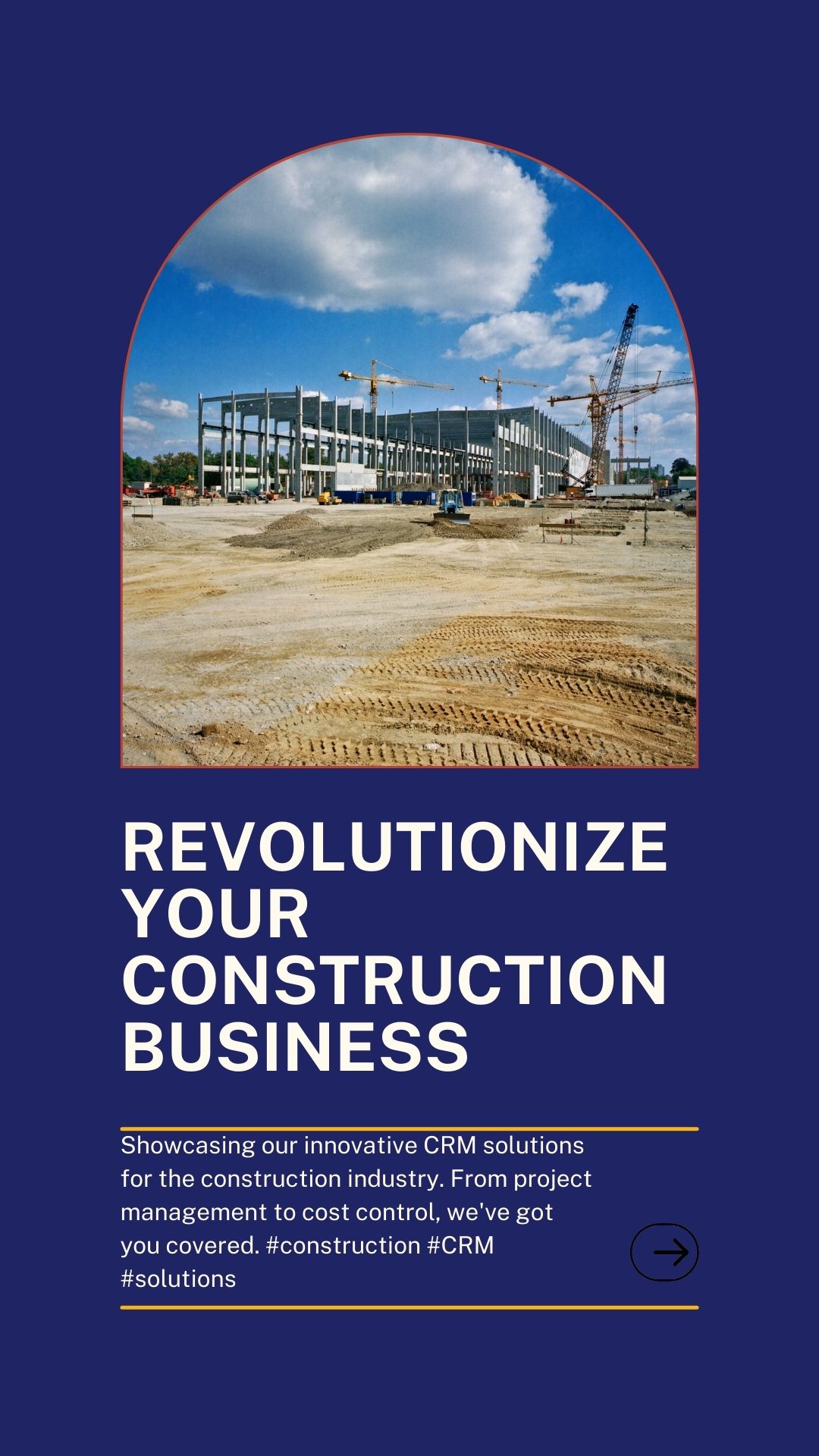
Predicting Customer Lifetime Value using Vector Databases transcends traditional analytics, providing a multi-dimensional view of customer interactions and transactions.
This advanced approach empowers businesses across various industries to not only identify their most valuable customers but also to strategize effectively in capturing and enhancing this value.
In an era where personalized customer experiences and efficient resource allocation are paramount, leveraging vector-based CLV predictions is indispensable for maximizing profitability and sustaining competitive advantage.