Enhancing Text Classification with Embeddings: A Leap in Understanding and Categorization
Content:
- Introduction
- The Evolution of Text Classification
- Role of Embeddings in Text Classification
- Business Applications
- Advantages of Using Embeddings in Classification
The Evolution of Text Classification
Text classification is a fundamental task in natural language processing (NLP), involving the categorization of text into predefined groups or labels.
Traditional methods rely heavily on keyword matching and frequency-based techniques, often missing the context and deeper semantic meaning.
The advent of Embeddings has revolutionized this field, offering a way to capture the nuanced meanings and relationships in text, thus significantly improving classification accuracy.
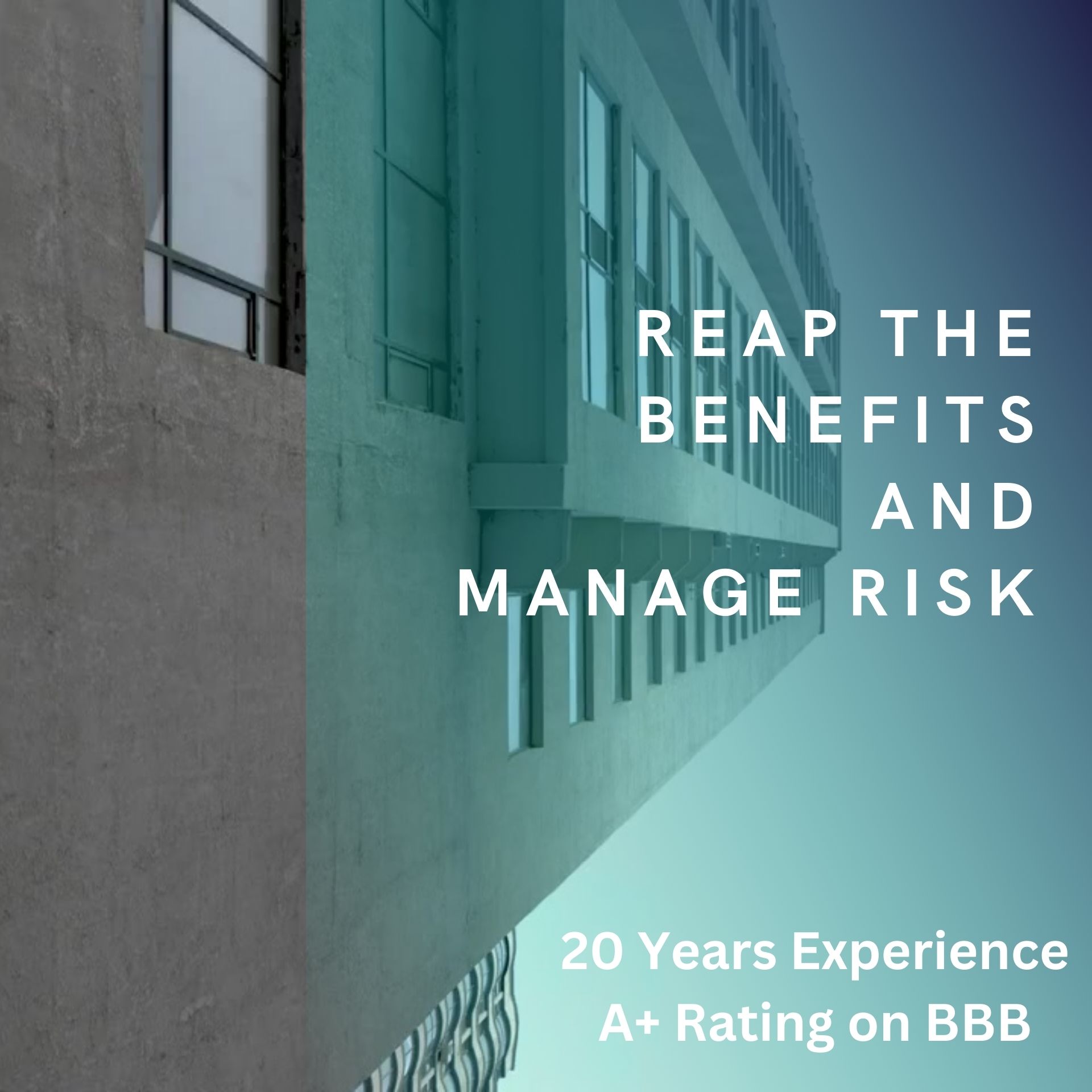
Role of Embeddings in Text Classification
Embeddings provide a vectorized representation of text, where each word, phrase, or document is mapped to Vectors in a continuous, high-dimensional space.
These vector representations capture more than just the superficial aspects of the text; they encode contextual meanings, syntactic patterns, and semantic relationships.
In text classification, these Embeddings allow for the grouping and categorization of text based not only on explicit content but also on underlying themes and contexts.
Business Applications
-
Customer Feedback Analysis:
Companies can classify customer feedback into categories such as complaints, suggestions, or compliments, allowing for targeted responses and improvements.
-
Email Sorting and Prioritization:
Businesses can use Embeddings to automatically classify incoming emails into categories like urgent, informational, or spam, thereby improving efficiency in communication management.
-
Social Media Monitoring:
Embeddings can classify social media posts by sentiment or topic, helping businesses in reputation management and market analysis.
-
Content Moderation:
Online platforms can automatically categorize user-generated content for appropriate categorization or flagging, ensuring community guidelines are upheld.
-
Legal Document Sorting:
Law firms and legal departments can classify documents by relevance, type, or subject matter, streamlining research and case preparation.
Advantages of Using Embeddings in Classification
-
Contextual Awareness:
Unlike traditional keyword-based approaches, Embeddings understand the context, enabling more nuanced classifications.
-
Handling of Ambiguity:
Embeddings are effective in distinguishing between different meanings of the same word in different contexts.
-
Scalability and Adaptability:
They can easily adapt to new, unseen categories or changes in language use over time, without the need for extensive reprogramming.
-
Improved Accuracy:
By capturing deeper semantic meanings, Embeddings provide more accurate and relevant classification results.
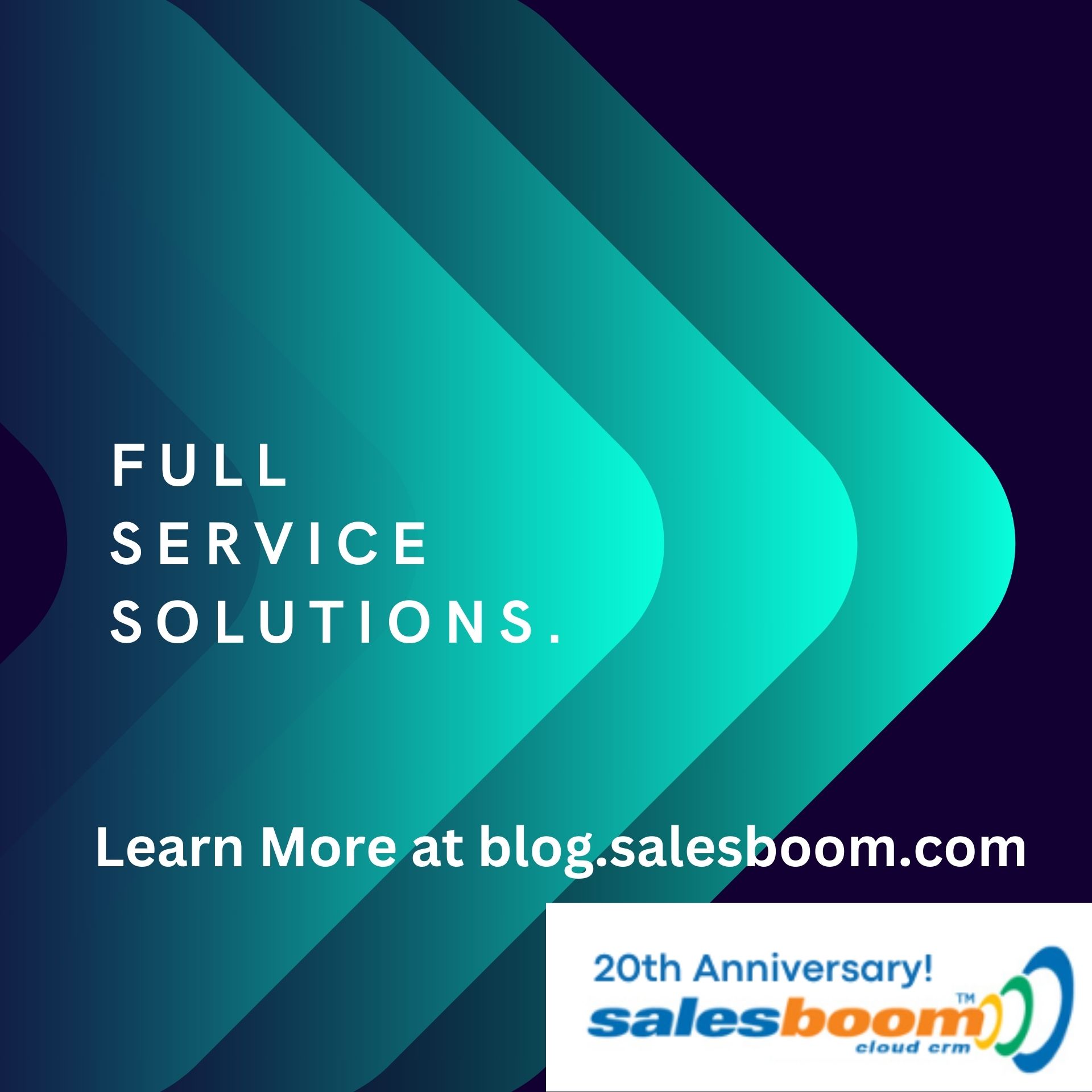
Embeddings have transformed the landscape of text classification, enabling a deeper and more contextually rich categorization of text.
This advancement empowers businesses to gain more insightful, accurate, and actionable outcomes from their text data.
Whether it's managing customer communications, moderating content, or analyzing market trends, the application of Embeddings in text classification provides a critical edge in harnessing the full potential of textual information in the digital age.